Friday 25 March 2016
Statistics of coincidences: Ben Geen case revisited (ABC)
In November 2014 I reported on the case of nurse Ben Geen who was convicted in 2006 for murdering 2 patients and seriously harming 15 others. I had been asked to produce an expert report on the 'statistical coincidences' in the case for the Criminal Cases Review Board.
Now a 30-minute documentary on the case presented by Joel Werner is to be aired on Australia's national radio station ABC on 28 March. In the programme (which you can listen to in full from the links at the top of the ABC page) I present a lay summary of the statistical argument (from minutes 16:30 to 21:34).
Norman Fenton
Saturday 19 March 2016
Turning poorly structured data into intelligent Bayesian Network models for medical decision support
Medical data is very often badly structured, incomplete and inconsistent. This limits our ability to generate useful models for prediction and decision support if we rely purely on machine learning techniques. That means we need to exploit expert knowledge at various model development stages. This problem - which is common in many application domains - is tackled in a paper** published in the latest issue of Artificial Intelligence in Medicine.
The paper describes a rigorous and repeatable method for building effective Bayesian Network (BN) models from complex data - much of which comes in unstructured and incomplete responses by patients from questionnaires and interviews. Such data inevitably contains repetitive, redundant and contradictory responses; without expert knowledge learning a BN model from the data alone is especially problematic where we are interested in simulating causal interventions for risk management. The novelty of this work is that it provides a rigorous consolidated and generalised framework that addresses the whole life-cycle of BN model development. The method is validated using data from forensic psychiatry. The resulting BN models demonstrate competitive to superior predictive performance against the data-driven state-of-the-art models. More importantly, the resulting BN models go beyond improving predictive accuracy and into usefulness for risk management through intervention, and enhanced decision support in terms of answering complex clinical questions that are based on unobserved evidence.
The method is applicable to any application domain involving large-scale decision analysis based on such complex and unstructured information. It challenges decision scientists to reason about building models based on what information is really required for inference, rather than based on what data is available. Hence, it forces decision scientists to use available data in a much smarter way.
**The full reference for the paper is:
Constantinou, A. C., Fenton, N., Marsh, W., & Radlinski, L. (2016). "From complex questionnaire and interviewing data to intelligent Bayesian Network models for medical decision support".Artificial Intelligence in Medicine, Vol 67 pages 75-93. DOI http://dx.doi.org/10.1016/j.artmed.2016.01.002
For those who do not have access to the journal a pre-publication draft can be downloaded: http://constantinou.info/downloads/papers/complexBN.pdf
Thursday 10 March 2016
A Bayesian network to determine optimal strategy for Spurs' success
As a committed Spurs fan I have spent the last few months salivating at the club's sudden and unexpected rise and the prospect of them winning their first league title since 1961. By mid-February they were clear favourites to win the Premier League title. However, in my view, the challenge was compromised by the team becoming overstretched by playing too many matches in a short space of time. In particular, I felt that their involvement in the Europa League was an unnecessary distraction and burden. When I expressed these views on a Spurs online forum (backed up with some data showing consistent under-performance during periods when they were involved in the Europa League) I got heavily criticised by other fans who said it was important to try to win every competition.
Having simultaneously been involved in research discussions about the use of decisions in Bayesian networks, I decided to build a small model in AgenaRisk to resolve the dilemma once and for all. I have written up the results of the analysis here. The model can be downloaded from here.
In summary, there were 4 strategic options available to Spurs' manager Mauricio Pochettino at the time I started to do the analysis:
- Focus on Premier League
- Focus on Premier League and FA Cup
- Focus on Premier League and Europa League
- Focus on all three competitions
Downloads:
- Norman Fenton "To focus on winning one competition or try to win more: Using a Bayesian network to help decide the optimum strategy for a football club", March 2016
- Bayesian network model for the paper
Tuesday 8 March 2016
Anthony Constantinou presents BAYES-KNOWLEDGE work in Parliament
![]() |
Anthony Constantinou and his poster |
Updated 8 March 2016
Anthony Constantinou was selected to present his work (summarised in this poster) on the BAYES-KNOWLEDGE project in Parliament on 7 March 2016 as part of the SET for Britain awards 2016 This was the Press Release from Parliament:
Dr Anthony Constantinou, 31, a Post-Doctoral Researcher at Queen Mary University of London, hailing from Limassol, Cyprus, is attending Parliament to present his mathematics research to a range of politicians and a panel of expert judges, as part of SET for Britain on Monday 7 March.Although Anthony did not win one of the final prizes his entry was highly praised by the judges and many MPs who he spoke with.
Anthony’s poster on research about Decision Systems which are based on probabilistic graphical models for uncertainty quantification and risk management, will be judged against dozens of other scientists’ research in the only national competition of its kind. Anthony is funded as part of the BAYES-KNOWLEDGE project (http://bayes-knowledge.com/).
Anthony was shortlisted from hundreds of applicants to appear in Parliament.
On presenting his research in Parliament, he said, “This is a great opportunity to demonstrate the art of intelligent decision making, which is merely based on identifying the best possible set of actions to be taken on the basis of trade-offs in an effort to achieve some objectives; then having to explain why an objective has not been met! Having applied these methods to a diverse range of real-world application domains, spanning from forensics and medical sciences to sports and gambling market efficiency, I do hope I can keep the audience entertained.”
Stephen Metcalfe MP, Chairman of the Parliamentary and Scientific Committee, said:
“This annual competition is an important date in the parliamentary calendar because it gives MPs an opportunity to speak to a wide range of the country’s best young researchers.
“These early career engineers, mathematicians and scientists are the architects of our future and SET for Britain is politicians’ best opportunity to meet them and understand their work.”
Anthony’s research has been entered into the Mathematical Sciences session of the competition, which will end in a gold, silver and bronze prize-giving ceremony.
Judged by leading academics, the gold medalist receives £3,000, while silver and bronze receive £2,000 and £1,000 respectively.
The Parliamentary and Scientific Committee runs the event in collaboration with the Royal Academy of Engineering, the Royal Society of Chemistry, the Institute of Physics, the Royal Society of Biology, The Physiological Society and the Council for the Mathematical Sciences, with financial support from Essar, the Clay Mathematics Institute, Warwick Manufacturing Group (WMG), the Institute of Biomedical Science, the Bank of England and the Society of Chemical Industry.
Anthony about to enter the Houses of Parliament |
Anthony with Nick Clegg and David Cameron! |
Anthony with poster |
![]() |
The prize giving bit with Science Minister Joe Johnson (5th from right) |
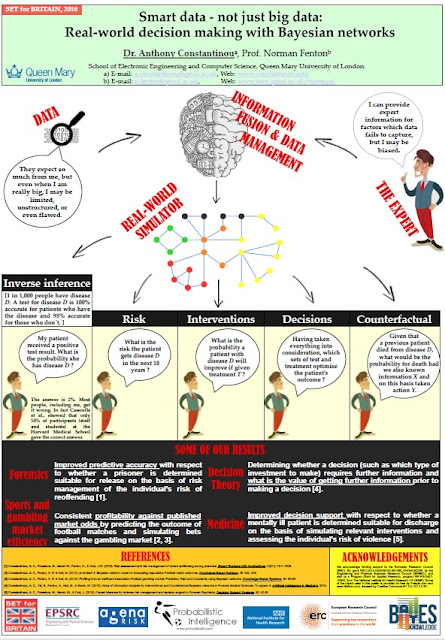
Improving Bayesian networks by learning from similar ones
A major challenge of the BAYES-KNOWLEDGE project is about how to build useful and accurate Bayesian network (BN) models for decision support when there is little relevant data. Much of what we have done involves exploiting expert judgment. But my colleagues Yun Zhou and Tim Hospedales have developed a so-called 'tranfer learning' method that enables us to leverage data from different but related problems. Suppose, for example, we have a BN model for a particular medical diagnostic problem that we built based on limited data and expert judgment in the UK. But suppose also that a model for the same (or very similar) diagnostic problem has been developed in the USA based on a much larger data set. Some assumptions in the US model will be different to the UK (such as the population demographics or particular testing methods) but much of the underlying pathology will be the same. The challenge is to understand and exploit the heterogeneous relatedness of the models.
The result of this work has just been published in an article in the Elsevier journal Expert Systems with Applications. Elsevier have provided free access to the article until April 24, 2016:
http://authors.elsevier.com/a/1SfM-3PiGT01St
The article describes a new transfer learning algorithm for improved BN parameter learning, and the experimental results demonstrate its superiority compared to other state-of-the-art parameter transfer methods. The method is applied to a real-world medical case study, namely the problem of trauma care (a problem for which our team had initially developed a decision support BN model in collaboration with UK medics).
Full reference for the new article:
Zhou, Y., Hospedales, T., Fenton, N. E. (2016), "When and where to transfer for Bayes net parameter learning", Expert Systems with Applications. 55, 361-373
http://dx.doi.org/10.1016/j.eswa.2016.02.011.
Subscribe to:
Posts (Atom)