Bayes Knowledge: Smart data – not big data
Friday, 21 December 2018
Final blog posting
As the BAYES-KNOWLEDGE project has now successfully completed all relevant news about the research from this and related projects will be posted either to the probability and risk blog or the Risk and Informations Management blog. There is also relevant material posted on the blog for the book.
Thursday, 20 December 2018
Review of “The Book of Why" by Pearl and Mackenzie
Judea Pearl and Dana Mackenzie: “The Book of Why: The New Science of Cause and Effect”, Basic Books, 2018. ISBN: 9780465097609
www.basicbooks.com/titles/judea-pearl/the-book-of-why/9780465097609/We have finally completed a detailed review of this important and outstanding book - the review will hopefully be published in the journal Artificial Intelligence. But a preprint of the full review is now available.
Some excerpts from the review:
- Judea Pearl, a Turing Award prize winner, is a true giant of the field of computer science and artificial intelligence. The Turing award is the highest distinction in computer science; i.e., the Nobel Prize of computing. To say that his new book with Dana Mackenzie is timely is, in our view, an understatement. Coming from somebody of his stature and being written for a general audience (unlike his previous books), means that the concerns we have held about both the limitations of solely data driven approaches to artificial intelligence (AI) and the need for a causal approach, will finally reach a very broad audience.
- According to Pearl, the state of the art in AI today is merely a ‘souped-up’ version of what machines could already do a generation ago: find hidden regularities in a large set of data. “All the impressive achievements of deep learning amount to just curve fitting”, he said recently.
- In Chapter 1, the core message about the need for causal models is underpinned by what Pearl calls “The Ladder of Causation”, which is then used to orient the ideas presented throughout the book. Pearl’s ladder of causation suggests that there are three steps to achieving true AI. .... Pearl also characterises these three steps on the ladder as 1) ‘seeing’; 2) ‘doing’; and 3) ‘imagining’.
- One of the reasons ‘deep learning’ has been so successful is that many problems can be solved by optimisation alone without the need to even consider advancing to rungs in the ladder of causation beyond the first. These problems include machine vision and machine listening, natural language processing, robot navigation, as well as other problems that fall within the areas of clustering, pattern recognition and anomaly detection. Big data in these cases is clearly very important and the advances being made using deep learning are undoubtedly impressive, but Pearl convincingly argues that they are not AI.
- There is much excellent material in this book but, for us, the two key messages are: 1) “True AI” cannot be achieved by data and curve fitting alone, since causal representation of the underlying problems is also required to answer “what-if” questions, and 2) Randomized control trials are not the only ‘valid’ method for determining causal effects.
For the full review see:
Review of: Judea Pearl and Dana Mackenzie: “The Book of Why: The New Science of Cause and Effect”, Basic Books, 2018 DOI: https://doi.org/10.13140/RG.2.2.27512.49925, by Norman Fenton, Martin Neil, and Anthony Constantinou
Thursday, 29 November 2018
AI for healthcare requires ‘smart data’ rather than ‘big data’
Norman Fenton gave a talk titled AI for healthcare requires ‘smart data’ rather than ‘big data’ to medics at the Royal London Hospital on 27 November. He explained the background and context for the PAMBAYESIAN project.
Norman's Powerpoint presentation
Norman's Powerpoint presentation
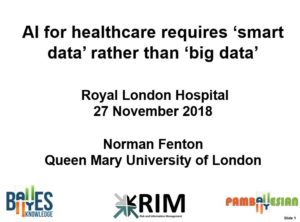
Thursday, 15 November 2018
Book Launch at the Turing Institute
Some photos from last night's book launch event at The Turing Institute
More photos
Norman Fenton and Martin Neil |
More photos
Tuesday, 13 November 2018
Book Launch event at The Turing Institute
On 14 November 2018 Norman Fenton and Martin Neil are hosting a
reception at The Turing Institute to celebrate the launch of the Second
Edition of their book "Risk Assessment and Decision Analysis with
Bayesian Networks".
Slide show of the book
A small number of places remain for people to register for the reception
Book Blog
Slide show of the book
A small number of places remain for people to register for the reception
Book Blog
Sunday, 7 October 2018
New research published in IEEE Transactions makes building accurate Bayesian networks easier
One of the biggest practical challenges in building Bayesian network (BN) models for decision support and risk assessment is to define the probability tables for nodes with multiple parents. Consider the following example:
To define the probability table for the node "Attack carried out" we have to define probability values for each possible combination of the states of the parent nodes, i.e., for all the entries of the following table.
That is 16 values (although, since the columns must sum to one we only really have to define 8).
When data are sparse - as in examples like this - we must rely on judgment from domain experts to elicit these values. Even for a very small example like this, such elicitation is known to be highly error-prone. When there are more parents (imagine there are 20 different terrorist cells) or more states other than "False" and "True", then it becomes practically infeasible. Numerous methods have been proposed to simplify the problem of eliciting such probability tables. One of the most popular methods - “noisy-OR”- approximates the required relationship in many real-world situations like the above example. BN tools like AgenaRisk implement the noisy-OR function making it easy to define even very large probability tables. However, it turns out that in situations where the child node (in the example this is the node "Attack carried out") is observed to be "False", the noisy-OR function fails to properly capture the real world implications. It is this weakness that is both clarified and resolved in the following two new papers.
Hence the first paper provides a 'complete solution' but requires software like AgenaRisk for its implementation, while the second paper provides a simple approximate solution.
Acknowledgements: The research was supported by the European Research Council under project, ERC-2013-AdG339182 (BAYES_KNOWLEDGE); the Leverhulme Trust under Grant RPG-2016-118 CAUSAL-DYNAMICS; Intelligence Advanced Research Projects Activity (IARPA), to the BARD project (Bayesian Reasoning via Delphi) of the CREATE programme under Contract [2017-16122000003]. and Agena Ltd for software support. We also acknowledge the helpful recommendations and comments of Judea Pearl, and the valuable contributions of David Lagnado (UCL) and Nicole Cruz (Birkbeck).
In any given week a terrorist organisation may or may not carry out an attack. There are several independent cells in this organisation for which it may be possible in any week to determine heightened activity. If it is known that there is no heightened activity in any of the cells, then an attack is unlikely. However, for any cell if it is known there is heightened activity then there is a chance an attack will take place. The more cells known to have heightened activity the more likely an attack is.In the case where there are three terrorist cells, it seems to reasonable to assume the BN structure here:
To define the probability table for the node "Attack carried out" we have to define probability values for each possible combination of the states of the parent nodes, i.e., for all the entries of the following table.
That is 16 values (although, since the columns must sum to one we only really have to define 8).
When data are sparse - as in examples like this - we must rely on judgment from domain experts to elicit these values. Even for a very small example like this, such elicitation is known to be highly error-prone. When there are more parents (imagine there are 20 different terrorist cells) or more states other than "False" and "True", then it becomes practically infeasible. Numerous methods have been proposed to simplify the problem of eliciting such probability tables. One of the most popular methods - “noisy-OR”- approximates the required relationship in many real-world situations like the above example. BN tools like AgenaRisk implement the noisy-OR function making it easy to define even very large probability tables. However, it turns out that in situations where the child node (in the example this is the node "Attack carried out") is observed to be "False", the noisy-OR function fails to properly capture the real world implications. It is this weakness that is both clarified and resolved in the following two new papers.
- Noguchi, T., Fenton, N. E., & Neil, M. (2018). "Addressing the Practical Limitations of Noisy-OR using Conditional Inter-causal Anti-Correlation with Ranked Nodes". IEEE Transactions on Knowledge and Data Engineering DOI: 10.1109/TKDE.2018.2873314 (This is the pre-publication version)
- Fenton, N. E., Noguchi, T. & Neil, M, (2018). "An extension to the noisy-OR function to resolve the “explaining away” deficiency for practical Bayesian network problems", IEEE Transactions on Knowledge and Data Engineering, under review
Hence the first paper provides a 'complete solution' but requires software like AgenaRisk for its implementation, while the second paper provides a simple approximate solution.
Acknowledgements: The research was supported by the European Research Council under project, ERC-2013-AdG339182 (BAYES_KNOWLEDGE); the Leverhulme Trust under Grant RPG-2016-118 CAUSAL-DYNAMICS; Intelligence Advanced Research Projects Activity (IARPA), to the BARD project (Bayesian Reasoning via Delphi) of the CREATE programme under Contract [2017-16122000003]. and Agena Ltd for software support. We also acknowledge the helpful recommendations and comments of Judea Pearl, and the valuable contributions of David Lagnado (UCL) and Nicole Cruz (Birkbeck).
Wednesday, 26 September 2018
Bayesian networks for trauma prognosis
There is an excellent online resource produced by Barbaros Yet that summarises the results of collaboration between the Risk and Information Management research group at Queen Mary and the Trauma Sciences Unit, Barts and the London School of Medicine and Dentistry. This work focused on developing Bayesian network (BN) models to improve decision support for trauma patients.
The website not only describes two BN models in detail (one for predicting acute traumatic coagulopathy in early stage of trauma care and one for predicting the outcomes of traumatic lower extremities with vascular injuries) but allows you to run the models in real time showing summary risk calculations after you enter observations about a patient.
The models are powered by AgenaRisk.
Links:
- http://traumamodels.com/
- Perkins ZB, Yet B, Glasgow S, Marsh DWR, Tai NRM, Rasmussen TE (2018). “Long-term, patient centered outcomes of Lower Extremity Vascular Trauma”, Journal of Trauma and Acute Surgery. DOI:10.1097/TA.0000000000001956
- Yet B, Perkins ZB, Tai NR, and Marsh DWR (2017). “Clinical Evidence Framework for Bayesian Networks” Knowledge and Information Systems, 50(1), pp.117-143.DOI:10.1007/s10115-016-0932-1
- Perkins ZB, Yet B, Glasgow S, Cole E, Marsh W, Brohi K, Rasmussen TE, Tai NRM (2015). “Meta-analysis of prognostic factors for amputation following surgical repair of lower extremity vascular trauma” British Journal of Surgery, 12 (5), pp. 436-450. DOI:10.1002/bjs.9689
- Yet B, Perkins ZB, Rasmussen TE et al.(2014). Combining data and meta-analysis to build Bayesian networks for clinical decision support. J Biomed Inform vol. 52, 373-385. http://dx.doi.org/10.1016/j.jbi.2014.07.018 http://qmro.qmul.ac.uk/xmlui/handle/123456789/23055
- Perkins ZB, Yet B, Glasgow S, Cole E, Marsh W, Brohi K,
Rasmussen TE, Tai NRM (2015). “Meta-analysis of prognostic factors for
amputation following surgical repair of lower extremity vascular trauma”
British Journal of Surgery, 12 (5), pp. 436-450. DOI:10.1002/bjs.9689
- Yet B, Perkins ZB, Rasmussen TE, Tai NR, and Marsh DWR
(2014). “Combining Data and Meta-analysis to Build Bayesian Networks for
Clinical Decision Support” Journal of Biomedical Informatics , 52, pp.373-385. DOI:10.1016/j.jbi.2014.07.018
- Yet B, Perkins Z, Fenton N et al.(2014). Not just data: a method for improving prediction with knowledge. J Biomed Inform vol. 48, 28-37. http://dx.doi.org/10.1016/j.jbi.2013.10.012
- Yet B, Perkins Z, Tai N et al.(2014). Explicit evidence for prognostic Bayesian network models. Stud Health Technol Inform vol. 205, 53-57. http://dx.doi.org/10.3233/978-1-61499-432-9-53
- Perkins Z, Yet B, Glasgow S et al. (2013). EARLY PREDICTION OF TRAUMATIC COAGULOPATHY USING ADMISSION CLINICAL VARIABLES. SHOCK. vol. 40, 25-25.
Subscribe to:
Posts (Atom)