Friday, 21 December 2018
Final blog posting
As the BAYES-KNOWLEDGE project has now successfully completed all relevant news about the research from this and related projects will be posted either to the probability and risk blog or the Risk and Informations Management blog. There is also relevant material posted on the blog for the book.
Thursday, 20 December 2018
Review of “The Book of Why" by Pearl and Mackenzie
Judea Pearl and Dana Mackenzie: “The Book of Why: The New Science of Cause and Effect”, Basic Books, 2018. ISBN: 9780465097609
www.basicbooks.com/titles/judea-pearl/the-book-of-why/9780465097609/We have finally completed a detailed review of this important and outstanding book - the review will hopefully be published in the journal Artificial Intelligence. But a preprint of the full review is now available.
Some excerpts from the review:
- Judea Pearl, a Turing Award prize winner, is a true giant of the field of computer science and artificial intelligence. The Turing award is the highest distinction in computer science; i.e., the Nobel Prize of computing. To say that his new book with Dana Mackenzie is timely is, in our view, an understatement. Coming from somebody of his stature and being written for a general audience (unlike his previous books), means that the concerns we have held about both the limitations of solely data driven approaches to artificial intelligence (AI) and the need for a causal approach, will finally reach a very broad audience.
- According to Pearl, the state of the art in AI today is merely a ‘souped-up’ version of what machines could already do a generation ago: find hidden regularities in a large set of data. “All the impressive achievements of deep learning amount to just curve fitting”, he said recently.
- In Chapter 1, the core message about the need for causal models is underpinned by what Pearl calls “The Ladder of Causation”, which is then used to orient the ideas presented throughout the book. Pearl’s ladder of causation suggests that there are three steps to achieving true AI. .... Pearl also characterises these three steps on the ladder as 1) ‘seeing’; 2) ‘doing’; and 3) ‘imagining’.
- One of the reasons ‘deep learning’ has been so successful is that many problems can be solved by optimisation alone without the need to even consider advancing to rungs in the ladder of causation beyond the first. These problems include machine vision and machine listening, natural language processing, robot navigation, as well as other problems that fall within the areas of clustering, pattern recognition and anomaly detection. Big data in these cases is clearly very important and the advances being made using deep learning are undoubtedly impressive, but Pearl convincingly argues that they are not AI.
- There is much excellent material in this book but, for us, the two key messages are: 1) “True AI” cannot be achieved by data and curve fitting alone, since causal representation of the underlying problems is also required to answer “what-if” questions, and 2) Randomized control trials are not the only ‘valid’ method for determining causal effects.
For the full review see:
Review of: Judea Pearl and Dana Mackenzie: “The Book of Why: The New Science of Cause and Effect”, Basic Books, 2018 DOI: https://doi.org/10.13140/RG.2.2.27512.49925, by Norman Fenton, Martin Neil, and Anthony Constantinou
Thursday, 29 November 2018
AI for healthcare requires ‘smart data’ rather than ‘big data’
Norman Fenton gave a talk titled AI for healthcare requires ‘smart data’ rather than ‘big data’ to medics at the Royal London Hospital on 27 November. He explained the background and context for the PAMBAYESIAN project.
Norman's Powerpoint presentation
Norman's Powerpoint presentation
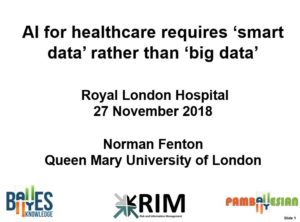
Thursday, 15 November 2018
Book Launch at the Turing Institute
Some photos from last night's book launch event at The Turing Institute
More photos
Norman Fenton and Martin Neil |
More photos
Tuesday, 13 November 2018
Book Launch event at The Turing Institute
On 14 November 2018 Norman Fenton and Martin Neil are hosting a
reception at The Turing Institute to celebrate the launch of the Second
Edition of their book "Risk Assessment and Decision Analysis with
Bayesian Networks".
Slide show of the book
A small number of places remain for people to register for the reception
Book Blog
Slide show of the book
A small number of places remain for people to register for the reception
Book Blog
Sunday, 7 October 2018
New research published in IEEE Transactions makes building accurate Bayesian networks easier
One of the biggest practical challenges in building Bayesian network (BN) models for decision support and risk assessment is to define the probability tables for nodes with multiple parents. Consider the following example:
To define the probability table for the node "Attack carried out" we have to define probability values for each possible combination of the states of the parent nodes, i.e., for all the entries of the following table.
That is 16 values (although, since the columns must sum to one we only really have to define 8).
When data are sparse - as in examples like this - we must rely on judgment from domain experts to elicit these values. Even for a very small example like this, such elicitation is known to be highly error-prone. When there are more parents (imagine there are 20 different terrorist cells) or more states other than "False" and "True", then it becomes practically infeasible. Numerous methods have been proposed to simplify the problem of eliciting such probability tables. One of the most popular methods - “noisy-OR”- approximates the required relationship in many real-world situations like the above example. BN tools like AgenaRisk implement the noisy-OR function making it easy to define even very large probability tables. However, it turns out that in situations where the child node (in the example this is the node "Attack carried out") is observed to be "False", the noisy-OR function fails to properly capture the real world implications. It is this weakness that is both clarified and resolved in the following two new papers.
Hence the first paper provides a 'complete solution' but requires software like AgenaRisk for its implementation, while the second paper provides a simple approximate solution.
Acknowledgements: The research was supported by the European Research Council under project, ERC-2013-AdG339182 (BAYES_KNOWLEDGE); the Leverhulme Trust under Grant RPG-2016-118 CAUSAL-DYNAMICS; Intelligence Advanced Research Projects Activity (IARPA), to the BARD project (Bayesian Reasoning via Delphi) of the CREATE programme under Contract [2017-16122000003]. and Agena Ltd for software support. We also acknowledge the helpful recommendations and comments of Judea Pearl, and the valuable contributions of David Lagnado (UCL) and Nicole Cruz (Birkbeck).
In any given week a terrorist organisation may or may not carry out an attack. There are several independent cells in this organisation for which it may be possible in any week to determine heightened activity. If it is known that there is no heightened activity in any of the cells, then an attack is unlikely. However, for any cell if it is known there is heightened activity then there is a chance an attack will take place. The more cells known to have heightened activity the more likely an attack is.In the case where there are three terrorist cells, it seems to reasonable to assume the BN structure here:
To define the probability table for the node "Attack carried out" we have to define probability values for each possible combination of the states of the parent nodes, i.e., for all the entries of the following table.
That is 16 values (although, since the columns must sum to one we only really have to define 8).
When data are sparse - as in examples like this - we must rely on judgment from domain experts to elicit these values. Even for a very small example like this, such elicitation is known to be highly error-prone. When there are more parents (imagine there are 20 different terrorist cells) or more states other than "False" and "True", then it becomes practically infeasible. Numerous methods have been proposed to simplify the problem of eliciting such probability tables. One of the most popular methods - “noisy-OR”- approximates the required relationship in many real-world situations like the above example. BN tools like AgenaRisk implement the noisy-OR function making it easy to define even very large probability tables. However, it turns out that in situations where the child node (in the example this is the node "Attack carried out") is observed to be "False", the noisy-OR function fails to properly capture the real world implications. It is this weakness that is both clarified and resolved in the following two new papers.
- Noguchi, T., Fenton, N. E., & Neil, M. (2018). "Addressing the Practical Limitations of Noisy-OR using Conditional Inter-causal Anti-Correlation with Ranked Nodes". IEEE Transactions on Knowledge and Data Engineering DOI: 10.1109/TKDE.2018.2873314 (This is the pre-publication version)
- Fenton, N. E., Noguchi, T. & Neil, M, (2018). "An extension to the noisy-OR function to resolve the “explaining away” deficiency for practical Bayesian network problems", IEEE Transactions on Knowledge and Data Engineering, under review
Hence the first paper provides a 'complete solution' but requires software like AgenaRisk for its implementation, while the second paper provides a simple approximate solution.
Acknowledgements: The research was supported by the European Research Council under project, ERC-2013-AdG339182 (BAYES_KNOWLEDGE); the Leverhulme Trust under Grant RPG-2016-118 CAUSAL-DYNAMICS; Intelligence Advanced Research Projects Activity (IARPA), to the BARD project (Bayesian Reasoning via Delphi) of the CREATE programme under Contract [2017-16122000003]. and Agena Ltd for software support. We also acknowledge the helpful recommendations and comments of Judea Pearl, and the valuable contributions of David Lagnado (UCL) and Nicole Cruz (Birkbeck).
Wednesday, 26 September 2018
Bayesian networks for trauma prognosis
There is an excellent online resource produced by Barbaros Yet that summarises the results of collaboration between the Risk and Information Management research group at Queen Mary and the Trauma Sciences Unit, Barts and the London School of Medicine and Dentistry. This work focused on developing Bayesian network (BN) models to improve decision support for trauma patients.
The website not only describes two BN models in detail (one for predicting acute traumatic coagulopathy in early stage of trauma care and one for predicting the outcomes of traumatic lower extremities with vascular injuries) but allows you to run the models in real time showing summary risk calculations after you enter observations about a patient.
The models are powered by AgenaRisk.
Links:
- http://traumamodels.com/
- Perkins ZB, Yet B, Glasgow S, Marsh DWR, Tai NRM, Rasmussen TE (2018). “Long-term, patient centered outcomes of Lower Extremity Vascular Trauma”, Journal of Trauma and Acute Surgery. DOI:10.1097/TA.0000000000001956
- Yet B, Perkins ZB, Tai NR, and Marsh DWR (2017). “Clinical Evidence Framework for Bayesian Networks” Knowledge and Information Systems, 50(1), pp.117-143.DOI:10.1007/s10115-016-0932-1
- Perkins ZB, Yet B, Glasgow S, Cole E, Marsh W, Brohi K, Rasmussen TE, Tai NRM (2015). “Meta-analysis of prognostic factors for amputation following surgical repair of lower extremity vascular trauma” British Journal of Surgery, 12 (5), pp. 436-450. DOI:10.1002/bjs.9689
- Yet B, Perkins ZB, Rasmussen TE et al.(2014). Combining data and meta-analysis to build Bayesian networks for clinical decision support. J Biomed Inform vol. 52, 373-385. http://dx.doi.org/10.1016/j.jbi.2014.07.018 http://qmro.qmul.ac.uk/xmlui/handle/123456789/23055
- Perkins ZB, Yet B, Glasgow S, Cole E, Marsh W, Brohi K,
Rasmussen TE, Tai NRM (2015). “Meta-analysis of prognostic factors for
amputation following surgical repair of lower extremity vascular trauma”
British Journal of Surgery, 12 (5), pp. 436-450. DOI:10.1002/bjs.9689
- Yet B, Perkins ZB, Rasmussen TE, Tai NR, and Marsh DWR
(2014). “Combining Data and Meta-analysis to Build Bayesian Networks for
Clinical Decision Support” Journal of Biomedical Informatics , 52, pp.373-385. DOI:10.1016/j.jbi.2014.07.018
- Yet B, Perkins Z, Fenton N et al.(2014). Not just data: a method for improving prediction with knowledge. J Biomed Inform vol. 48, 28-37. http://dx.doi.org/10.1016/j.jbi.2013.10.012
- Yet B, Perkins Z, Tai N et al.(2014). Explicit evidence for prognostic Bayesian network models. Stud Health Technol Inform vol. 205, 53-57. http://dx.doi.org/10.3233/978-1-61499-432-9-53
- Perkins Z, Yet B, Glasgow S et al. (2013). EARLY PREDICTION OF TRAUMATIC COAGULOPATHY USING ADMISSION CLINICAL VARIABLES. SHOCK. vol. 40, 25-25.
Tuesday, 4 September 2018
It's finally arrived...
Still waiting to get our own copies of the second edition of the book,
but one of our PhD students just received his copy, so it is real! Note that sample chapters and lots of other resources are available on the book's blog. The first edition (published Dec 2012) has 437 Google scholar citations,
and many dozens of 5-star reviews on Amazon.
Friday, 24 August 2018
Second Edition of our book to be published 28 August 2018
From the back cover of the Second Edition:
************************************************
"The single most important book on Bayesian methods for decision analysts" —Doug Hubbard (author in decision sciences and actuarial science)
"The book provides sufficient motivation and examples (as well as the mathematics and probability where needed from scratch) to enable readers to understand the core principles and power of Bayesian networks." —Judea Pearl (Turing award winner)
"The lovely thing about Risk Assessment and Decision Analysis with Bayesian Networks is that it holds your hand while it guides you through this maze of statistical fallacies, p-values, randomness and subjectivity, eventually explaining how Bayesian networks work and how they can help to avoid mistakes.” —Angela Saini (award-winning science journalist, author & broadcaster)Since the first edition of this book published, Bayesian networks have become even more important for applications in a vast array of fields. This second edition includes new material on influence diagrams, learning from data, value of information, cybersecurity, debunking bad statistics, and much more. Focusing on practical real-world problem-solving and model building, as opposed to algorithms and theory, it explains how to incorporate knowledge with data to develop and use (Bayesian) causal models of risk that provide more powerful insights and better decision making than is possible from purely data-driven solutions.
Features
- Provides all tools necessary to build and run realistic Bayesian network models
- Supplies extensive example models based on real risk assessment problems in a wide range of application domains provided; for example, finance, safety, systems reliability, law, forensics, cybersecurity and more
- Introduces all necessary mathematics, probability, and statistics as needed
- Establishes the basics of probability, risk, and building and using Bayesian network models, before going into the detailed applications
************************************************
Sample chapters are available on the book's website
Wednesday, 25 July 2018
Updating Prior Beliefs Based on Ambiguous Evidence
Suppose two nations, North Bayesland and South Bayesland are independently testing new missile technology. Each has made six detonation attempts: North Bayesland has been successful once and South Bayesland four times. You observe another detonation on the border between the two countries but cannot determine the source. Based only on the provided information:
- What is the probability that North (or South) Bayesland is the source of this missile?
- What is your best estimate of the propensity for success of North and South Bayesland after this latest observation (i.e. the probability, for each nation, that a future missile they launch will detonate)?
Our paper "Updating Prior Beliefs Based on Ambiguous Evidence", which was accepted at the prestigious 40th Annual Meeting of the Cognitive Science Society (CogSci 2018) in Madison, Wisconsin, addresses this problem. Stephen Dewitt (former QMUL PhD student) is presenting the paper on 27 July.
First of all the normative answer to Question 1 - based on simple Bayesian reasoning - is 20% for North Bayesland and 80% for South Bayesland. But Question 2 is much more complex because we cannot assume the small amount of data on previous detonation attempts represents a 'fixed' propensity of success (the normative Bayesian solution requires a non-trivial Bayesian network that models our uncertainty about the success propensities).
Based on experiments involving 250 paid participants, we discovered two types of errors in the answers.
- There was a ‘double updating’ error: individuals appear to first use their prior beliefs to interpret the evidence, then use the interpreted form of the evidence, rather than the raw form, when updating.
- We found an error where individuals convert from a probabilistic representation of the evidence to a categorical one and use this representation when updating.
The full paper details and pdf (also available here)
Dewitt, S, Lagnado, D, Fenton N. E (2018), "Updating Prior Beliefs Based on Ambiguous Evidence", CogSci 2018, Madison Wisconsin, 25-28 July 2018
This research is based upon work supported in part by the Office of the Director of National Intelligence (ODNI), Intelligence Advanced Research Projects Activity (IARPA), under Contract [2017-16122000003]. The views and conclusions contained herein are those of the authors and should not be interpreted as necessarily representing the official policies, either expressed or implied, of ODNI, IARPA, or the U.S. Government. The U.S. Government is authorized to reproduce and distribute reprints for governmental purposes notwithstanding any copyright annotation therein. Funding was also provided by the ERC project ERC-2013-AdG339182-BAYES_KNOWLEDGE and the Leverhulme Trust project RPG-2016-118 CAUSAL-DYNAMICS.
UPDATE: Stephen Dewitt presenting the paper in Madison:
Saturday, 14 July 2018
How to handle uncertain priors in Bayesian reasoning
In the classic simple Bayesian problem we have:
But what if there is uncertainty about the prior probabilities (i.e. the 1 in a 1000, the 5% and 0%). Maybe the 5% means 'anywhere between 0 and 10%'. Maybe the 1 in a 1000 means we only saw it once in 1000 people. This new technical report explains how to properly incorporate uncertainty about the priors using a Bayesian Network.
- a hypothesis H (such as 'person has specific disease') with a prior probability (say 1 in a 1000) and
- evidence E (such as a test result which may be positive or negative for the disease) for which we know the probability E given H (for example the probability of a false positive is 5% and the probability of a false negative is 0%).
But what if there is uncertainty about the prior probabilities (i.e. the 1 in a 1000, the 5% and 0%). Maybe the 5% means 'anywhere between 0 and 10%'. Maybe the 1 in a 1000 means we only saw it once in 1000 people. This new technical report explains how to properly incorporate uncertainty about the priors using a Bayesian Network.
Fenton NE, "Handling Uncertain Priors in Basic Bayesian Reasoning", July 2018, DOI 10.13140/RG.2.2.16066.89280
Friday, 13 July 2018
How much do we trust academic 'experts'?
Queen Mary has released the following press release about our new paper: Osman, M., Fenton, N. E., Pilditch, T., Lagnado, D. A., & Neil. M. (2018). "Who do we trust on social policy interventions", to appear next week in Basic and Applied Social Psychology. The preprint of the paper is here. There are already a number of press reports on it (see below).
People trust scientific experts more than the government even when the evidence is outlandish
Members of the public in the UK and US have far greater trust in scientific experts than the government, according to a new study by Queen Mary University of London. In three large scale experiments, participants were asked to make several judgments about nudges - behavioural interventions designed to improve decisions in our day-to-day lives.Press reports:
The nudges were introduced either by a group of leading scientific experts or a government working group consisting of special interest groups and policy makers. Some of the nudges were real and had been implemented, such as using catchy pictures in stairwells to encourage people to take the stairs, while others were fictitious and actually implausible like stirring coffee anti-clockwise for two minutes to avoid any cancerous effects.
The study, published in Basic and Applied Social Psychology, found that trust was higher for scientists than the government working group, even when the scientists were proposing fictitious nudges. Professor Norman Fenton, from Queen Mary’s School of Electronic Engineering and Computer Science, said: “While people judged genuine nudges as more plausible than fictitious nudges, people trusted some fictitious nudges proposed by scientists as more plausible than genuine nudges proposed by government. For example, people were more likely trust the health benefits of coffee stirring than exercise if the former was recommended by scientists and the latter by government.”
The results also revealed that there was a slight tendency for the US sample to find the nudges more plausible and more ethical overall compared to the UK sample. Lead author Dr Magda Osman from Queen Mary’s School of Biological and Chemical Sciences, said: “In the context of debates regarding the loss of trust in experts, what we show is that in actual fact, when compared to a government working group, the public in the US and UK judge scientists very favourably, so much so that they show greater levels of trust even when the interventions that are being proposed are implausible and most likely ineffective. This means that the public still have a high degree of trust in experts, in particular, in this case, social scientists.” She added: “The evidence suggests that trust in scientists is high, but that the public are sceptical about nudges in which they might be manipulated without them knowing. They consider these as less ethical and trust the experts proposing them less with nudges in which they do have an idea of what is going on.”
Nudges have become highly popular decision-support methods used by governments to help in a wide range of areas such as health, personal finances, and general wellbeing. The scientific claim is that to help people make better decisions regarding their lifestyle choices, and those that improve the welfare of the state, it is potentially effective to subtly change the framing of the decision-making context, which makes the option which maximises long term future gains more prominent. In essence the position adopted by nudge enthusiasts is that poor social outcomes are often the result of poor decision-making, and in order to address this, behavioural interventions such as nudges can be used to reduce the likelihood of poor decisions being made in the first place.
Dr Osman said: “Overall, the public make pretty sensible judgments, and what this shows is that people will scrutinize the information they are provided by experts, so long as they are given a means to do it. In other words, ask the questions in the right way, and people will show a level of scrutiny that is often not attributed to them. So, before there are strong claims made about public opinion about experts, and knee-jerk policy responses to this, it might be worth being a bit more careful about how the public are surveyed in the first place.”
- The Independent "People trust scientific experts far more than politicians, study shows"
- Health Magazine "For Americans in Science They Trust"
- The London Economic "People trust scientific experts more than the government even when the evidence is outlandish
- The Times:In science we trust even if it's poppycock:
- The Daily Record: Stirred by science:
Tuesday, 3 July 2018
How Bayesian Networks are pioneering the ‘smart data’ revolution
The July issue of Open Access Government has a 2-page article summarising our recent research and tool developments on Bayesian networks. A high-res pdf article of the article can be found here or here.
Thursday, 28 June 2018
Guilty Until Proven Innocent: The Crisis in Our Justice System
As mentioned in my previous posting I was invited by Jon Robins (the Justice Gap) to speak at the third meeting of the All-Party Parliamentary Group on Miscarriages of Justice, hosted by Barry Sheerman MP, in the House of Commons on 25 June 2018. The meeting was based around the launch of Jon Robins' outstanding new book "Guilty Until Proven Innocent: The Crisis in Our Justice System". Other speakers were: Michael Mansfield QC and lawyer Matt Foot who have been involved in many of the cases described in the book; Waney Squier the world-renowned neuropathologist who suffered for being one of the few medical experts to question the mainstream medical guidelines on 'shaken baby syndrome'; Gloria Morrison who spoke about the problems of Joint Enterprise relevant to some of the cases; and Liam Allan and Eddie Gilfoyle who spoke about their own experiences (theirs are are two of the cases discussed in the book). It was a very powerful and informative meeting which was very well attended (with many having to stand for the full two hours)
I have now written a detailed review of the book which includes more about the House of Commons meeting. (Note an updated version which fixes some errors in the Researchgate version is available here)
See also
- Guilty until proven innocent: Book review (also available here)
- On the role of statistics in miscarriages of justice
- Statistics of coincidence: Ben Geen case revisited
- Ben Geen: another possible case of miscarriage of justice and misunderstanding of statistics
- Jon Robins, “Guilty Until Proven Innocent: The Crisis in Our Justice System”. Biteback Publishing, 2018. ISBN 978-1-78590-369-4
- Fenton, N. E. (2018). On the Role of Statistics in Miscarriages of Justice. In 3rd Meeting of the All-Party Parliamentary Group on Miscarriages of Justice. House of Commons, London 25 June 2018
- Review of the use of Bayes in the Law (pdf report)
- Barry George case: new insights on the evidence.
- Sally Clark case: another statistical oversight
Monday, 25 June 2018
On the Role of Statistics in Miscarriages of Justice
I have been invited by Jon Robins (the Justice Gap) to speak today at the third meeting of the All-Party Parliamentary Group on Miscarriages of Justice, hosted by Barry Sheerman MP, in the House of Commons Jon Robins will be talking about his outstanding new book "Guilty Until Proven Innocent: The Crisis in Our Justice System" at the event. The book includes a description of the Ben Geen case for which I provided a report to the Criminal Cases Review Commission in 2015 showing that the sequence of 'unusual events' at the Horton General Hospital (where Ben Geen worked as a nurse) was not especially unusual. (UPDATE: See report about the meeting, including detailed review of the book)
My short talk today focuses on the role of statistics in miscarriages of justice. A transcript of the talk can be found here.
Norman Fenton
See also
- Guilty until proven innocent
- Statistics of coincidence: Ben Geen case revisited
- Ben Geen: another possible case of miscarriage of justice and misunderstanding of statistics
- Jon Robins, “Guilty Until Proven Innocent: The Crisis in Our Justice System”. Biteback Publishing, 2018. ISBN 978-1-78590-369-4
- Fenton, N. E. (2018). On the Role of Statistics in Miscarriages of Justice. In 3rd Meeting of the All-Party Parliamentary Group on Miscarriages of Justice. House of Commons, London 25 June 2018
- Review of the use of Bayes in the Law (pdf report)
- Barry George case: new insights on the evidence.
- Sally Clark case: another statistical oversight
Friday, 22 June 2018
Bias in AI Algorithms
On 17 Jan 2018 multiple news sources (e.g. see here, here, and here) ran a story about a new research paper that claims to expose both the inaccuracies and racial bias in COMPAS - one of the most common algorithms used for parole and sentencing decisions to predict recidivism (i.e. whether or not a defendant will re-offend).
The research paper was written by the world famous computer scientist Hany Farid (along with a student Julia Dressel).
But the real story here is that the paper’s accusation of racial bias (specifically that the algorithm is biased against black people) is based on a fundamental misunderstanding of causation and statistics. The algorithm is no more ‘biased’ against black people than it is biased against white single parents, old people, people living in Beattyville Kentucky, or women called ‘Amber’. In fact, as we show in this brief article, if you choose any factor that correlates with poverty you will inevitably replicate the statistical ‘bias’ claimed in the paper. And if you accept the validity of the claims in the paper then you must also accept, for example, that a charity which uses poverty as a factor to identify and help homeless people is being racist because it is biased against white people (and also, interestingly, Indian Americans).
The fact that the article was published and that none of the media running the story realise that they are pushing fake news is what is most important here. Depressingly, many similar research studies involving the same kind of misinterpretation of statistics result in popular media articles that push a false narrative of one kind or another.
22 June 2018 Update: It turns out that now Microsoft is "developing a tool to help engineers catch bias in algorithms" This article also cites the case of the COMPAS software:
"...., which uses machine learning to predict whether a defendant will commit future crimes, was found to judge black defendants more harshly than white defendants."Interestingly, this latest news article about Microsoft does NOT refer to the 2018 Dressel and Fardi article but, rather, to an earlier 2016 article by Larson et al: https://www.propublica.org/article/how-we-analyzed-the-compas-recidivism-algorithm From a quick inspection it does seem to be a more comprehensive study than the flawed Dressel and Farid article. But my quick impression is that the same fundamental misunderstandings statistics/causality are there. Given the great degree of interest in AI/bias, and given also that we were unaware of the 2016 study, we plan to do an update to our unpublished paper.
Our article (5 pages): Fenton, N.E., & Neil, M. (2018). "Criminally Incompetent Academic Misinterpretation of Criminal Data - and how the Media Pushed the Fake News" http://dx.doi.org/10.13140/RG.2.2.32052.55680 Also available here.
The research paper: Dressel, J. & Farid, H. The accuracy, fairness, and limits of predicting recidivism. Sci. Adv. 4, eaao5580 (2018).
Thanks to Scott McLachlan for the tip off on this story.
See some previous articles on poor use of statistics:
Wednesday, 20 June 2018
New project: Bayesian Artificial Intelligence for Decision Making under Uncertainty
Anthony Constantinou - a lecturer based in the Risk and Information Management Group at Queen Mary University of London - has been awarded a prestigious 3-year EPSRC Fellowship Grant £475,818 in partnership with Agena Ltd to develop open-source software that will enable end-users to quickly and efficiently generate Bayesian Decision Networks (BDNs) for optimal real-world decision-making. BDNs are Bayesian Networks augmented with additional functionality and knowledge-based assumptions to represent decisions and associated utilities that a decision maker would like to optimize. BDNs are suitable for modelling real-world situations where we seek to discover the optimal decision path to maximise utilities of interest and minimise undesirable risk.
A full description of the project can be found here. The EPSRC announcement is here.
Links
Thursday, 24 May 2018
The limitations of machine learning
Readers of this and our other blogs will be aware that we have long been sceptical of the idea that 'big data' - coupled with clever machine learning algorithms - will be able to achieve improved decision-making and risk assessment as claimed (see links below). We believe that a smart data approach that combines expert judgment (including understanding of underlying causal mechanisms) with relevant data is required and that Bayesian Networks (BNs) provide an ideal formalism for doing this effectively.
Turing award winner Judea Pearl, who was the pioneer of BNs, has just published a new book "The Book of Why: The New Science of Cause and Effect", which delivers essentially this same message. And there is a great interview with Pearl in the Atlantic Magazine about the book and his current views. The article includes the following:
As he sees it, the state of the art in artificial intelligence today is merely a souped-up version of what machines could already do a generation ago: find hidden regularities in a large set of data. “All the impressive achievements of deep learning amount to just curve fitting,” he said recently.Read it all.
The interview also refers to the article "Human-Level Intelligence or Animal-Like Abilities?" by Adnan Darwiche. This is an outstanding paper (8 pages) that explains in more detail why we do not need to be over impressed by deep learning.
Links
- How a Pioneer of Machine Learning Became One of Its Sharpest Critics
- Pearl: "The Book of Why"
- Adnan Darwiche's article "Human-Level Intelligence or Animal-Like Abilities?"
- Pearl: "Theoretical Impediments to Machine Learning With Seven Sparks from the Causal Revolution"
- The problem with big data and machine learning
- A short story illustrating why pure machine learning (without expert input) may be doomed to fail and totally unnecessary (2 page pdf)
- Another machine learning fable: explains why pure machine learning for identifying credit risk may result in perfectly incorrect risk assessment (1 page pdf)
- Moving from big data and machine learning to smart data and causal modelling: a simple example from consumer research and marketing (7 page pdf)
![]() |
Norman gets his hands on Pearl's new book |
Friday, 18 May 2018
Probability and Statistics in Forensics
Never got round to posting this summary (it's the image above- click to enlarge) of our Isaac Newton Institute Cambridge Programme on Probability and Statistics in Forensic Science. The full version of the annual 2016-17 Report is here.
See also:
- Bayesian Networks and Argumentation in Evidence Analysis
- Bayes and the Law: what's been happening in Cambridge and how you can see it
- Recommendations for Dealing with Quantitative Evidence in Criminal Law
- Bayes and the Law: Cambridge event and new review paper
- Confusion over the Likelihood ratio
- The Bayesian networks mutual exclusivity problem
- Use of Bayes in the Netherlands Court of Appeal
Friday, 4 May 2018
Anthony Constantinou's football prediction system wins second spot in international competition
Anthony Constantinou |
QMUL lecturer Dr Anthony Constantinou of the RIM research group has come second in an international competition to produce the most accurate football prediction system. Moreover, the winners (whose predictive accuracy was only very marginally better) actually based their model on the previously published pi-ratings system of Constantinou and Fenton.
Anthony's model Dolores was developed for the International Machine Learning for Soccer Competition hosted by the Machine Learning journal.
All participants were provided with the results of matches from 52 different leagues around the world - with some missing data as part of the challenge. They had to produce a single model before the end of March 2017 that would be tested on its accuracy of predicting 206 future match outcomes from 26 different leagues, played from March 31 to April 9 in 2017.
Dolores was ranked 2nd with a predictive accuracy almost the same as the top ranked system (there was less than 1% error rate difference between the two; the error rate was nearly 120% lower than the participants ranked lowest among those that passed the basic criteria).
Dolores is designed to predict football match outcomes in one country by observing football matches in multiple other countries.It is based on a) dynamic ratings and b) Hybrid Bayesian Networks.
Unlike past academic literature which tends to focus on a single league or tournament, Dolores provides empirical proof that a model can make a good prediction for a match outcome between teams 𝑥 and 𝑦 even when the prediction is derived from historical match data that neither 𝑥 nor 𝑦 participated in. This implies that we can still predict, for example, the outcome of English Premier League matches, based on training data from Japan, New Zealand, Mexico, South Africa, Russia, and other countries in addition to data from the English Premier league.
The Machine Learning journal has published the descriptions of the highest ranked systems in its latest issue published online today. The full reference for Anthony's paper is:
Constantinou, A. (2018). Dolores: A model that predicts football match outcomes from all over the world. Machine Learning, 1-27, DOI: https://doi.org/10.1007/s10994-018-5703-7
The full published version can be viewed (for free) at https://rdcu.be/Nntp. An open access pre-publication version (pdf format) is available for download here.
This work was partly supported by the European Research Council (ERC), research project ERC-2013-AdG339182-BAYES_KNOWLEDGE
The DOLORES Hybrid Bayesian Network was built and run using the AgenaRisk software.
The full reference for the pi-ratings model (used by the competition's winning team) is:
Constantinou, A. C. & Fenton, N. E. (2013). Determining the level of ability of football teams by dynamic ratings based on the relative discrepancies in scores between adversaries. Journal of Quantitative Analysis in Sports. Vol. 9, Iss. 1, 37–50. DOI: http://dx.doi.org/10.1515/jqas-2012-0036See also:
Open access version here.
- Anthony's pi-football website.
- Explaining and predicting football results over an entire season
- Explaining Bayesian networks through a football management problem
- The problem with predicting football results
- A Bayesian network to determine optimal strategy for Spurs' success
- Proving referee bias with Bayesian networks
Monday, 30 April 2018
Bayesian Nets to Determine Impact of Agricultural Development Policy
An interesting paper - describing use of Bayesian nets to determine impact of agricultural development policy on household nutrition in Uganda - uses the new 'Value of Information' functionality developed in BAYES-KNOWLEDGE.
Full reference:
Cory W. Whitney Denis Lanzanova Caroline Muchiri Keith D. Shepherd Todd S. Rosenstock Michael Krawinkel John R. S. Tabuti Eike Luedeling (2018), "Probabilistic Decision Tools for Determining Impacts of Agricultural Development Policy on Household Nutrition", Earth's Future (Open Access) https://doi.org/10.1002/2017EF000765
Tuesday, 17 April 2018
Explaining Bayesian Networks through a football management problem
Today's Significance Magazine (the magazine of the Royal Statistical Society and the American Statistical Association) has published an article by Anthony Constantinou and Norman Fenton that explains, through the use of an example from football management, the kind of assumptions required to build useful Bayesian networks (BNs) for complex decision-making. The article highlights the need to fuse data with expert knowledge, and describes the challenges in doing so. It also explains why, for fully optimised decision-making, extended versions of BNs, called Bayesian decision networks, are required.
The published pdf (open source) is also available here and here.
Full article details:
Constantinou, A., Fenton, N.E, "Things to know about Bayesian networks", Significance, 15(2), 19-23 April 2018, https://doi.org/10.1111/j.1740-9713.2018.01126.x
Wednesday, 14 March 2018
Evidence based decision making turns knowledge into power
A nice 2-page article about our BAYES-KNOWLEDGE project is in the latest issue of EU Research Magazine Beyond the Horizon. A pdf version is here.
Tuesday, 6 March 2018
Two coins: one fair one biased
Alexander Bogolmony tweeted this problem:
If there is no reason to assume in advance that either coin is more likely to be the coin tossed once (i.e. the first coin) then all the (correct) solutions show that the first coin is more likely to be biased with a probability of 9/17 (=0.52941). Here is an explicit Bayesian network solution for the problem:
The above figure shows the result after entering the 'evidence' (i.e. one Head on the coin tossed once and two Heads on the coin tossed three times). The tables displayed are the conditional probability tables defined for the associated with the variables.
This model took just a couple of minutes to build in AgenaRisk and requires absolutely no manual calculations as the Binomial distribution is one of many functions pre-defined. The model (which can be run in the free version of AgenaRisk is here). The nice thing about this solution compared to the others is that it is much more easily extendible. It also shows the reasoning very clearly.
If there is no reason to assume in advance that either coin is more likely to be the coin tossed once (i.e. the first coin) then all the (correct) solutions show that the first coin is more likely to be biased with a probability of 9/17 (=0.52941). Here is an explicit Bayesian network solution for the problem:
The above figure shows the result after entering the 'evidence' (i.e. one Head on the coin tossed once and two Heads on the coin tossed three times). The tables displayed are the conditional probability tables defined for the associated with the variables.
This model took just a couple of minutes to build in AgenaRisk and requires absolutely no manual calculations as the Binomial distribution is one of many functions pre-defined. The model (which can be run in the free version of AgenaRisk is here). The nice thing about this solution compared to the others is that it is much more easily extendible. It also shows the reasoning very clearly.
Monday, 12 February 2018
An Improved Method for Solving Hybrid Influence Diagrams
Most decisions are made in the face of uncertain factors and outcomes. In a typical decision problem, uncertainties involve both continuous factors (e.g. amount of profit) and discrete factors (e.g. presence of a small number of risk events). Tools such as decision trees and influence diagrams are used to cope with uncertainty regarding decisions, but most implementations of these tools can only deal with discrete or discretized factors and ignore continuous factors and their distributions.
A paper just published in the International Journal of Approximate Reasoning presents a novel method that overcomes a number of these limitations. The method is able to solve decision problems with both discrete and continuous factors in a fully automated way. The method requires that the decision problem is modelled as a Hybrid Influence Diagrams, which is an extension of influence diagrams containing both discrete and continuous nodes, and solves it by using a state-of-the-art inference algorithm called Dynamic Discretization. The optimal policies calculated by the method are presented in a simplified decision tree.
The full reference is:
Acknowledgements: Part of this work was performed under the auspices of EU project ERC-2013-AdG339182-BAYES_KNOWLEDGE
A paper just published in the International Journal of Approximate Reasoning presents a novel method that overcomes a number of these limitations. The method is able to solve decision problems with both discrete and continuous factors in a fully automated way. The method requires that the decision problem is modelled as a Hybrid Influence Diagrams, which is an extension of influence diagrams containing both discrete and continuous nodes, and solves it by using a state-of-the-art inference algorithm called Dynamic Discretization. The optimal policies calculated by the method are presented in a simplified decision tree.
The full reference is:
Yet, B., Neil, M., Fenton, N., Dementiev, E., & Constantinou, A. (2018). "An Improved Method for Solving Hybrid Influence Diagrams". International Journal of Approximate Reasoning. DOI: 10.1016/j.ijar.2018.01.006 Preprint (open access) available here.UPDATE (22 Feb 2018): The full published version the paper is available online for free for 50 days here: https://authors.elsevier.com/c/1Wc6D,KD6ZG8y-
Acknowledgements: Part of this work was performed under the auspices of EU project ERC-2013-AdG339182-BAYES_KNOWLEDGE
Friday, 9 February 2018
Decision-making under uncertainty: computing "Value of Information"
Information gathering is a crucial part of decision making under uncertainty. Whether to collect additional information or not, and how much to invest for such information are vital questions for successful decision making. For example, before making a treatment decision, a physician has to evaluate the benefits and risks of additional imaging or laboratory tests and decide whether to ask for them. Value of Information (VoI) is a quantitative decision analysis technique for answering such questions based on a decision model. It is used to prioritise the parts of a decision model where additional information is expected to be useful for decision making.
However, computing VoI in decision models is challenging especially when the problem involves both discrete and continuous variables. A new paper in the IEEE Access journal illustrates a simple and practical approach that can calculate VoI using Influence Diagram models that contain both discrete and continuous variables. The proposed method can be applied to a wide variety of decision problems as most decisions can be modelled as an influence diagram, and many decision modelling tools, including Decision Trees and Markov models, can be converted to an influence diagram.
The full reference is:
Yet, B., Constantinou, A., Fenton, N., & Neil, M. (2018). Expected Value of Partial Perfect Information in Hybrid Models using Dynamic Discretization. IEEE Access. DOI: 10.1109/ACCESS.2018.2799527
Acknowledgements: Part of this work was performed under the auspices of EU project ERC-2013-AdG339182-BAYES_KNOWLEDGE, EPSRC project EP/P009964/1: PAMBAYESIAN, and ICRAF Contract No SD4/2012/214 issued to Agena.
Wednesday, 7 February 2018
Lawnmower v terrorist risk: the saga continues
![]() |
Kim Kardashian's tweet comparing risk from lawnmowers v terrorists triggered the award and debate |
Yesterday Significance Magazine (the magazine of the Royal Statistical Society and the American Statistical Association) published an article “Lawnmowers versus Terrorists” with the strapline:
The Royal Statistical Society’s first ‘International Statistic of the Year’ sparked plenty of online discussion. Here, Norman Fenton and Martin Neil argue against the choice of winner, while Nick Thieme writes in support.Our case, titled “A highly misleading view of risk”, was an edited version of a paper previously publicised in a blog post that itself followed up on original concerns raised by Nicholas Nassim Taleb about the RSS citation and the way it had been publicised. The ‘opposing’ case made by Nick Thieme was essentially a critique of our paper.
We have today published a response to Nick’s critique.
Links:
- Norman Fenton,Martin Neil,Nick Thieme "Lawnmowers versus terrorists" Significance Magazine Volume 15, Issue 1,February 2018 Pages 12–13
- Norman Fenton and Martin Neil We have today also published a response to Nick’s critique: http://dx.doi.org/10.13140/RG.2.2.30958.72002
- Are lawnmowers a greater risk than terrorists?
- On lawnmowers and terrorists again: the danger of using historical data alone for decision-making
Subscribe to:
Posts (Atom)